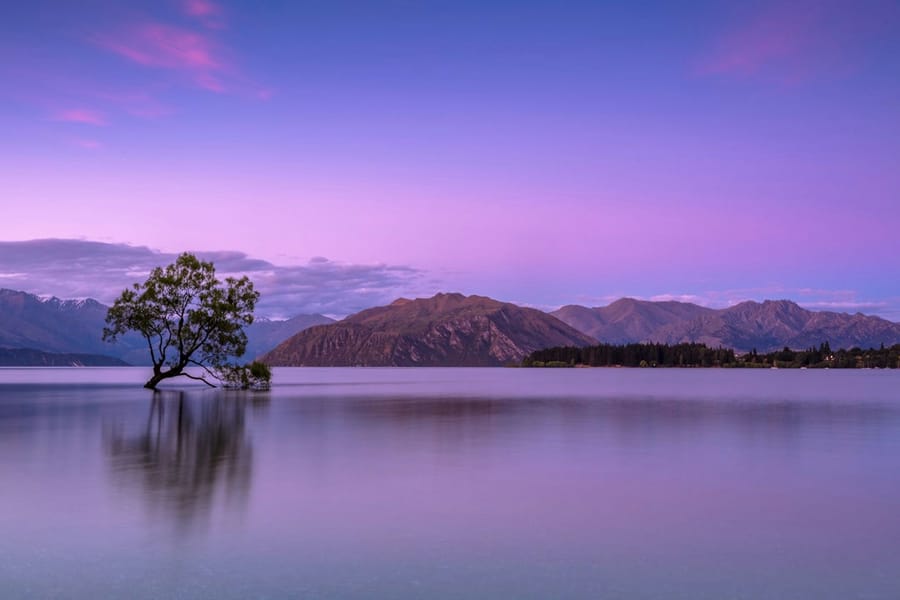
AI is changing the game for innovation at companies everywhere. When organizations use AI to generate ideas, develop concepts, and make better decisions, their creative processes take off. Think about this: people using ChatGPT-4 generate ideas 40x faster than traditional methods. That means pumping out 800 ideas per hour instead of just 20 without AI help.
This goes way beyond just saving time. AI-driven innovation creates a partnership where technology and human creativity work together. This lets teams come up with better ideas, evaluate options more thoroughly, and implement solutions faster than ever before.
What is AI-driven innovation?
AI-driven innovation uses smart computer systems to supercharge the creative process. While traditional approaches rely on human brainstorming sessions and manual evaluation, AI methods use machine learning, natural language processing, and predictive analytics to generate, refine, and implement new ideas.
The key idea here is what researchers call the "Both/And Principle." AI doesn't replace human creativity but amplifies it. The technology works like a thought partner, helping teams overcome biases, explore unusual solutions, and process mountains of information that would overwhelm any human.
When AI systems and human innovators team up, several things happen:
- Ideas get processed in parallel rather than one at a time
- Teams avoid groupthink and status-based filtering
- Different knowledge areas connect in ways humans might miss
- Teams can test ideas quickly without building physical prototypes
The technology behind all this keeps getting better. Today's systems use large language models for coming up with ideas, computer vision for refining designs, and specialized algorithms for solving specific problems. All these work together to make humans more creative.
Benefits and real-world applications
The benefits of AI-driven innovation extend far beyond simple efficiency gains. Organizations implementing AI in their creative processes are experiencing:
- Dramatically increased ideation capacity, with teams generating ideas at unprecedented speed
- Democratization of innovation across departments, regardless of formal creative training
- Enhanced idea evaluation through data-driven assessment rather than gut feeling
- Accelerated time-to-market by compressing multiple stages of the innovation pipeline
These advantages are transforming how businesses operate across multiple industries:
Fashion industry
Tommy Hilfiger employs AI-driven tools to analyze fashion trends, past collection performance, and social media data to create designs that better align with customer preferences. The technology processes vast amounts of visual and textual information to identify emerging patterns that human designers might miss, ensuring collections resonate with market demands.
Food industry
Waitrose created a top-selling dessert by using AI to analyze social media buzz, trending ingredients, and popular home-cooked dishes. Their innovation team leveraged the Tastewise platform to gather real-time insights from social media conversations, ingredient trends, and home cooking patterns, resulting in their classic creamy Basque cheesecake becoming a bestseller.
Supply chain optimization
Amazon's AI-driven supply chain uses machine learning to analyze data from various sources in real-time, predicting demand shifts with remarkable accuracy. The system integrates information from sales history, social media trends, economic indicators, and weather patterns to anticipate market changes before they occur, allowing for proactive inventory management.
Healthcare diagnostics
IBM Watson leverages AI algorithms to analyze extensive medical data, delivering evidence-based diagnostic insights that enhance patient outcomes. The platform processes medical literature, patient records, and clinical guidelines at a scale impossible for human physicians, identifying potential diagnoses that might otherwise be overlooked.
Fast food operations
KFC achieved a forecasting accuracy rate of approximately 95% using AI, significantly reducing food waste and optimizing staffing. Their implementation of the MacromatiX solution has transformed operational efficiency by matching inventory with consumer demand and ensuring appropriate staffing levels during peak periods.
Cybersecurity and manufacturing
AI-driven cybersecurity systems can transform threat detection from days to seconds, while predictive maintenance in manufacturing identifies equipment issues before catastrophic failures occur. Pfizer aggregates cybersecurity data sources to cut analysis times dramatically, while General Electric monitors equipment health in factories to predict potential failures before they happen.
The AI-driven innovation process
When you bring AI into your innovation workflow, you create a structured but flexible approach to solving problems creatively. Here's how that typically works:
Idea generation and discovery
AI systems excel at coming up with new ideas through semantic search and predictive modeling. Large language models can generate hundreds of potential solutions by mixing existing knowledge in surprising ways. Specialized algorithms can find gaps in current market offerings by spotting patterns in consumer data.
AI really shines when exploring the "adjacent possible" โ ideas that are new but still feasible given current constraints. By systematically searching through possible solutions, AI can find opportunities human teams might miss because of cognitive biases or limited attention.
Idea evaluation and scoring
After generating a bunch of potential innovations, AI systems can objectively assess which ones have the best chance of success. Machine learning algorithms evaluate new concepts against historical performance data, competitive landscape, and resource requirements.
These evaluations look at multiple factors at once:
- Market size and growth potential
- Technical complexity and timeline
- Resource needs and ROI projections
- Alignment with company strategy and capabilities
This data-driven approach helps teams prioritize the most promising innovations for development, reducing personal biases in the selection process.
Development and prototyping
During development, AI speeds up prototyping through automated design iteration and simulation. Generative design tools can produce dozens of variations based on core parameters. Digital twins let teams test virtual prototypes before spending resources on physical production.
This rapid iteration cycle dramatically shrinks the traditional development timeline, letting organizations refine concepts quickly based on continuous feedback.
Testing and validation
AI enhances testing through automated performance analysis and feedback interpretation. Machine learning algorithms can spot patterns in user responses that might suggest improvements. Natural language processing can extract actionable insights from qualitative feedback.
Implementation frameworks
Several structured methodologies have emerged to guide organizations in implementing AI throughout their innovation process:
AI-Centered Design Thinking This framework embeds AI tools at each stage of the traditional design thinking process:
- Empathize: AI analyzes customer data to identify unstated needs
- Define: AI clusters problems to reveal core challenges
- Ideate: Generative AI produces diverse solution concepts
- Prototype: AI tools rapidly visualize ideas
- Test: Machine learning identifies patterns in user feedback
AI-Adaptive Three-Horizons Framework This approach uses AI to manage innovations across different timeframes:
- Horizon 1 (immediate): AI optimizes existing products through A/B testing
- Horizon 2 (emerging): AI identifies market trends and predicts customer needs
- Horizon 3 (future): Generative AI explores radical new business models
AI-Enabled Open Innovation This methodology analyzes contributions from external sources:
- Scans patents, research papers, and social media
- Identifies complementary technologies and potential partners
- Discovers emerging solutions to targeted problems
- Expands innovation inputs beyond internal resources
AI workflow automation creates adaptive workflows that learn and improve over time, unlike traditional rule-based systems that blindly follow instructions. This adaptive approach ensures that innovation processes evolve based on outcomes, continuously optimizing for better results.
Implementing AI-driven innovation in your organization
Getting AI-driven innovation right takes thoughtful planning and alignment across your organization:
Assessment of innovation needs and opportunities
Start by finding specific innovation challenges where AI can make the biggest difference. Look for processes with clear metrics, enough available data, and significant upside if you could speed things up.
Focus areas might include:
- Product development cycles that could move faster with better iteration
- Marketing initiatives that need more personalized creative concepts
- Operational workflows with bottlenecks in decision-making
The goal is to identify high-leverage opportunities where your organization faces constraints that AI can help overcome.
Selecting the right AI tools and technologies
The AI tool landscape keeps evolving rapidly, with options ranging from general-purpose platforms to specialized solutions. Consider factors like:
- How well they integrate with your existing systems
- Whether they have features specific to your innovation needs
- How easily they'll scale as your innovation initiatives grow
- Whether non-technical team members can use them without help
Many organizations start with accessible generative AI tools like ChatGPT or Midjourney before investing in more specialized solutions tailored to their industry.
Building the necessary data infrastructure
Effective AI-driven innovation needs quality data inputs. Make sure you have:
- Enough historical data to train and validate AI models
- Real-time data pipelines to capture market signals
- Standardized data formats that AI systems can process
- Clear data governance policies that enable innovation while protecting sensitive information
This foundational infrastructure becomes the backbone of all your AI innovation initiatives, determining how effectively the technology can enhance your creative process.
Training teams and fostering adoption
The human element remains critical for successful implementation. Teams need both technical skills to work with AI tools and cultural readiness to embrace new ways of innovating.
Key training areas include:
- Prompt engineering to effectively communicate with AI systems
- Critical evaluation of AI-generated outputs
- Collaborative workflows that combine human and AI strengths
- Ethical considerations in AI-assisted innovation
Organizations often find value in creating cross-functional innovation teams that combine domain expertise with technical AI knowledge.
Measuring success and iterating
Set clear metrics to evaluate the impact of your AI-driven innovation initiatives:
- Idea volume and quality metrics
- Time-to-market acceleration
- Success rates of launched innovations
- Resource efficiency in the innovation process
These measurements provide feedback for continuous improvement, helping you refine your AI innovation approach over time.
Overcoming common challenges and resistance
Organizations typically run into several hurdles when implementing AI-driven innovation:
- Initial skepticism about AI's creative capabilities
- Concerns about job displacement among innovation teams
- Technical integration issues with existing systems
- Cultural resistance to changing established innovation processes
Addressing these challenges requires transparent communication about how AI augments rather than replaces human creativity, along with visible executive sponsorship of new approaches.
The bottom line
AI-driven innovation fundamentally changes how organizations tackle creative problem-solving. By combining human creativity with computational power, companies can generate more ideas, evaluate them more effectively, and implement solutions faster than ever before.
The organizations gaining a competitive edge today treat AI not as a separate initiative but as an integrated capability that enhances every stage of their innovation process. As AI technologies continue to evolve, their impact on innovation will only grow stronger, making now the perfect time for forward-thinking companies to begin their implementation journey.
For decision-makers considering next steps, start with a focused pilot in a high-value innovation area, measure the results carefully, and scale successful approaches across your organization. The transformative potential of AI-driven innovation is simply too significant to ignore.
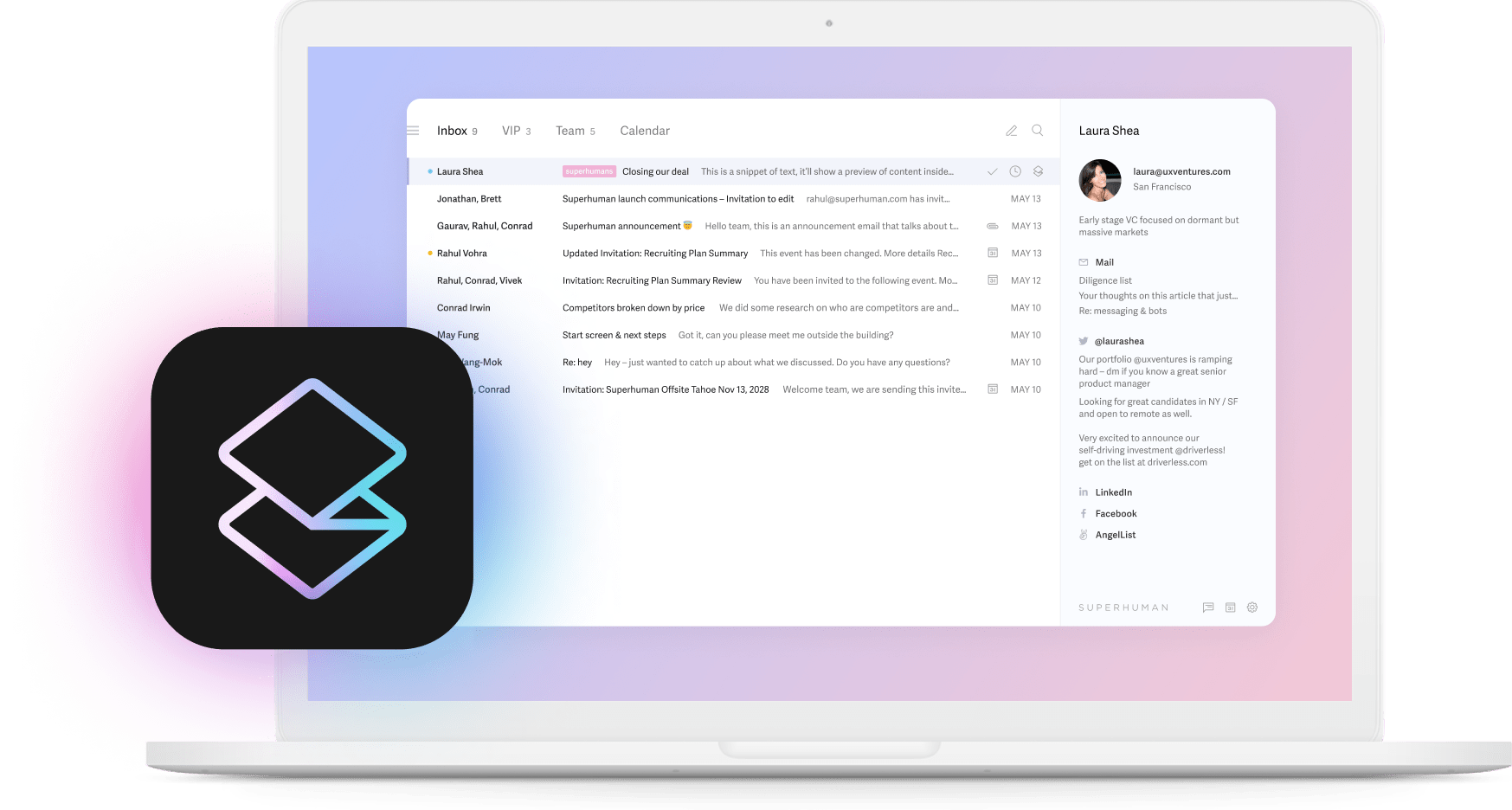
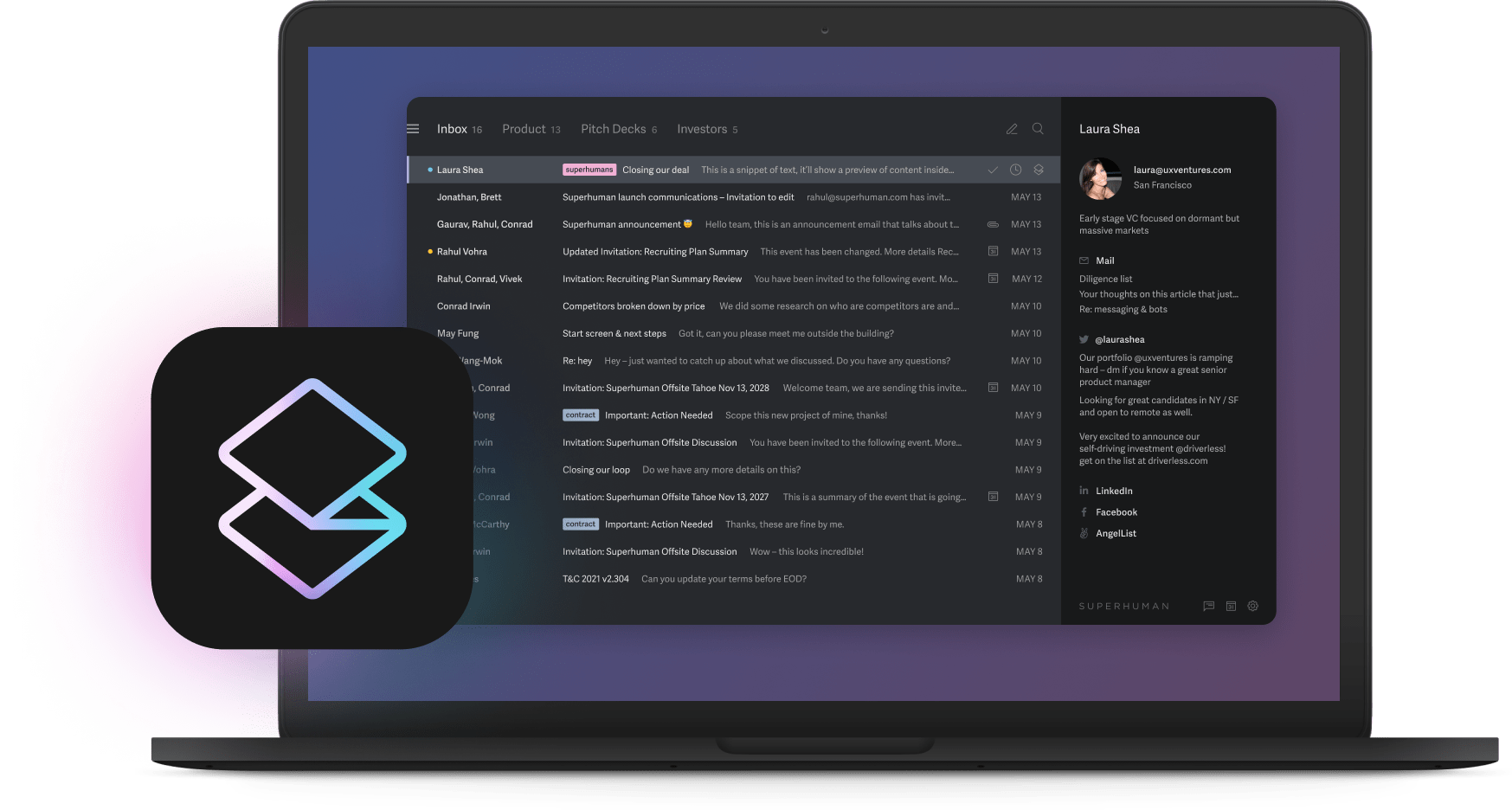