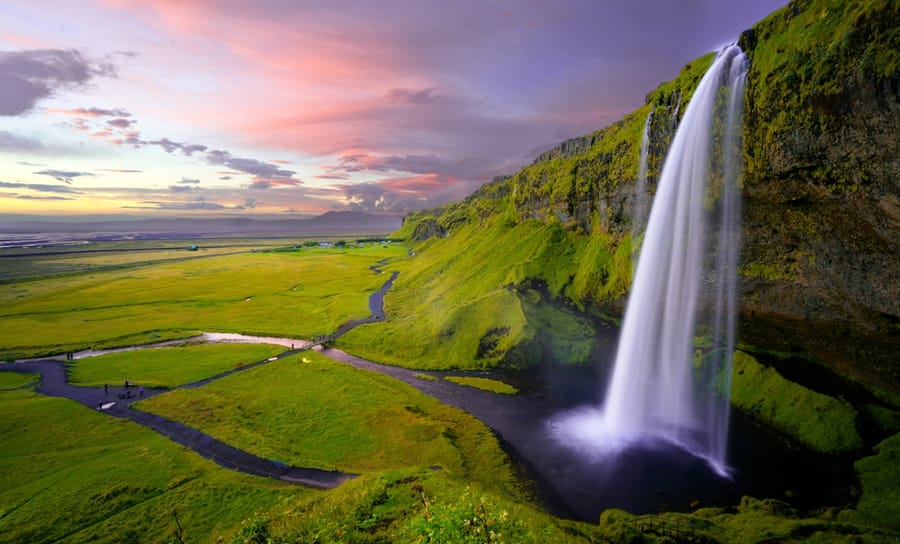
AI crashed into business decision making like a meteor. What started as faster data crunching turned into something nobody expected.
After talking with dozens of leaders who've implemented AI successfully, we've noticed a pattern. Nobody succeeds by trying to transform everything at once. The winners target specific decisions where better, faster choices create immediate impact. They build momentum through visible wins that matter to the business.
The decision revolution no one saw coming
The executive meeting hasn't disappeared. But what happens inside has completely changed.
Smart companies create partnerships between human judgment and machine intelligence. Humans keep strategic control while machines handle the analytical heavy lifting. This combination works because each contributes what the other lacks.
Three technologies power this shift:
- Machine learning algorithms that spot patterns humans miss
- Predictive analytics that show what's coming next
- Natural language processing that turns everyday communication into intelligence
Want to try this approach? Look for decisions that happen frequently, follow patterns, and currently create bottlenecks. Your email system already contains valuable signals about customer priorities, team alignment, and emerging issues. Connecting these signals to AI tools creates immediate insights without massive infrastructure changes.
Try SuperhumanThe 7 core benefits of AI in decision making
1. Speed that compounds into competitive advantage
In competitive markets, speed creates compound advantages. Fast decisions mean more learning cycles and more chances to adjust when things change.
Teams using AI tools respond to emails 12 hours faster on average. This acceleration extends beyond communication to core business decisions. Retailers spot demand shifts and adjust inventory before competitors notice. Financial firms execute trades when market conditions hit specific thresholds.
Sales teams gain particular advantages from faster decision making. While competitors wait days to qualify leads or respond to inquiries, AI-enhanced teams prioritize opportunities instantly based on communication patterns and buyer signals.
2. When machines don't get tired or play favorites
We humans make wildly inconsistent decisions. We overweight recent information. We favor evidence that confirms what we already believe. We make different choices depending on whether we're tired, hungry, or distracted.
AI in decision-making eliminates these inconsistencies. Healthcare organizations use this to improve diagnostic accuracy. Financial institutions reduce both fraud and false positives through more consistent transaction analysis.
Product teams use this capability to evaluate opportunities objectively. Instead of letting the loudest customer voices or most persuasive internal advocates drive roadmap decisions, they establish clear criteria and apply them consistently across all potential investments.
The accuracy advantage grows over time. As AI systems process more decisions and outcomes, they continuously refine their understanding of what works. This creates a virtuous cycle where each decision improves the quality of future ones.
The best implementations start with clear decision criteria that teams already use informally. Making these explicit allows AI to apply them consistently while still adapting to contextual factors that matter.
3. Processing everything, not just what fits on a dashboard
Most organizations still make decisions based on surprisingly small data samples. They analyze a handful of marketing campaigns, focus on a few customer segments, or optimize parts of operations in isolation.
AI in decision-making breaks this constraint by processing comprehensive datasets that human teams simply cannot manage. Marketing teams uncover subtle preference patterns across diverse customer groups. Supply chain managers identify optimization opportunities across entire distribution networks rather than local segments.
Implementing this benefit means connecting previously isolated data sources. When support tickets, product usage data, and sales conversations merge into a unified analysis environment, surprising patterns emerge that often challenge established assumptions.
4. Freedom from the decisions nobody wants to make
Every organization wastes significant resources on routine decisions that follow predictable patterns. Approval workflows. Resource allocation. Scheduling. Each consumes time better spent on higher-value activities.
Worker productivity increases by 66% when using AI tools. This productivity jump comes from eliminating low-value decisions while improving information quality for high-value ones.
The path to implementation starts with identifying which decisions happen most frequently. Quality control, resource allocation, and standard approvals often provide natural starting points. Success depends on clearly defining when decisions require human review versus when systems can proceed independently.
5. Catching problems while they're still small
Traditional risk management struggles with rapidly changing conditions. Historical patterns offer limited guidance when facing new situations.
AI approaches risk differently by continuously monitoring diverse signals. Energy companies spot equipment anomalies before failures occur. Financial institutions detect subtle transaction patterns that might indicate fraud before significant damage happens.
Risk teams implement this capability by establishing normal behavioral baselines, then detecting meaningful deviations. Effective approaches combine structured data analysis with natural language processing of communications, news feeds, and regulatory updates to provide early warning of emerging risks.
The most sophisticated implementations create dynamic risk profiles that evolve as conditions change. Rather than static models that fail during market shifts, these systems continuously recalibrate based on new information.
Most teams begin with a single, well-defined risk domain where outcomes can be clearly measured. This focused approach builds confidence while developing the organizational muscles needed for broader applications.
6. When everyone follows the same playbook
Most organizations struggle with consistency across teams, departments, and geographies. This inconsistency creates confusion, reduces trust, and undermines effectiveness.
AI acts as the great equalizer by applying identical standards to every situation. Whether evaluating loan applications, assessing vendor proposals, or prioritizing projects, consistent frameworks improve both outcomes and stakeholder satisfaction.
The essential first step involves making decision criteria explicit rather than relying on implicit knowledge. This transparency allows organizations to identify and address biases before implementing enhanced decision processes.
7. Seeing around corners before your competitors
Perhaps AI's most valuable contribution comes through improved long-term planning. Traditional approaches often fail to account for how multiple variables might interact under changing conditions.
Generative AI enhances decision-making by simulating multiple futures simultaneously. This transforms strategic planning from educated guessing into a more systematic, analytical process.
Strategic teams benefit from identifying critical uncertainties, then using AI to model different combinations of possibilities. Creating dashboards that highlight early warning signs for various scenarios helps organizations spot emerging patterns before competitors notice them.
The technology works by running thousands of simulations that would be impossible to calculate manually. Each simulation explores how different variables might interact under various conditions, creating a map of potential futures rather than a single prediction.
This approach doesn't eliminate uncertainty. It makes uncertainty manageable by identifying the factors that matter most and clarifying how different decisions might play out under various conditions.
Where AI is already reshaping industries
Finance and banking: from gut feel to precision
Financial institutions pioneered algorithmic decision making decades ago, but recent advances have dramatically expanded capabilities. Modern systems detect subtle fraud patterns by analyzing transaction sequences, timing patterns, and behavioral anomalies simultaneously.
Investment decisions benefit from natural language processing applied to earnings calls, regulatory filings, and market commentary. This context-rich approach identifies signals that purely numerical analysis would miss.
Credit decisions represent another crucial application. Traditional models often exclude qualified borrowers with limited credit histories. Newer approaches incorporate alternative data sources while maintaining appropriate risk controls.
Healthcare: when being right matters most
Physicians face impossible information management challenges. Medical knowledge doubles approximately every 73 days, far exceeding human capacity to stay current.
AI addresses this challenge by analyzing medical images, patient histories, and research literature simultaneously. Radiologists identify subtle abnormalities more consistently. Treatment planning improves through analysis of similar patient outcomes under various protocols.
Resource allocation becomes more patient-centered with AI systems optimizing staffing based on anticipated needs rather than rigid schedules, improving both care quality and operational efficiency.
Successful healthcare applications focus on augmenting clinical judgment rather than replacing it. Starting with narrowly defined use cases where outcomes can be clearly measured builds confidence for broader adoption.
Retail and e-commerce: knowing what customers want before they do
Retail success hinges on inventory decisions. Stock too little and you miss sales. Stock too much and margins collapse under clearance discounts.
Amazon employs AI throughout its supply chain, with algorithms predicting customer demand to position inventory proactively. This capability allows them to stock the right products in the right locations before customers even search for them.
Customer experiences improve through sophisticated personalization. Rather than simple product recommendations based on past purchases, AI analyzes browsing behavior, comparison patterns, and purchase timing to identify genuine customer intent.
Pricing optimization represents another high-impact application. AI systems analyze demand patterns, competitive positioning, and customer behavior to determine optimal price points that maximize both revenue and margins.
Manufacturing and supply chain: resilience by design
Manufacturing organizations face complex tradeoffs between efficiency, quality, and flexibility. AI helps balance these competing priorities through more sophisticated production planning.
Quality control reaches new levels of precision with AI-powered inspection systems. Computer vision detects subtle defects human inspectors might miss. Acoustic analysis identifies machinery problems before visible symptoms appear.
Supply chain resilience improves through continuous monitoring of potential disruption signals. Weather patterns, port congestion, supplier financial health, and transportation networks feed into dynamic risk assessments.
The real competitive edge isn't what you think
The transformation happening through AI in decision making goes well beyond automation. Companies gaining the biggest advantages aren't replacing human judgment. They're surrounding it with better information, delivered faster, with fewer blind spots.
For most organizations, the implementation journey begins by identifying communication bottlenecks and decision points where information overload creates delays. Email and messaging platforms often provide the quickest path to value, as these systems contain rich signals about priorities, opportunities, and emerging issues.
The businesses pulling ahead aren't attempting wholesale transformation. They target specific decisions where better outcomes create immediate value. This focused approach builds momentum through visible wins while developing the organizational capabilities needed for broader adoption.
As AI capabilities continue evolving, the gap between organizations that embrace these technologies and those that resist them will widen. The competitive advantage lies not in automation alone, but in creating a genuine partnership between human judgment and machine intelligence.
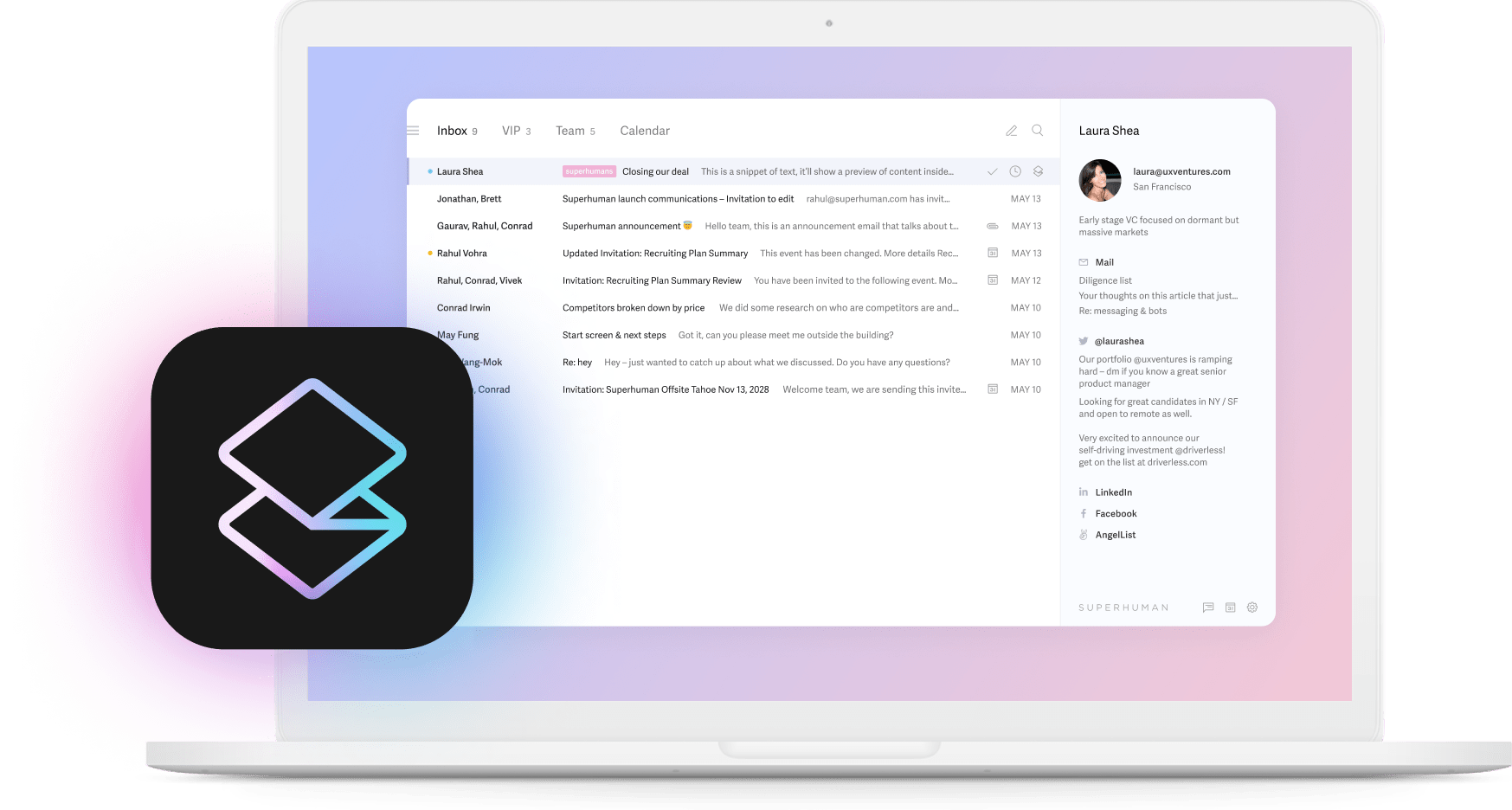
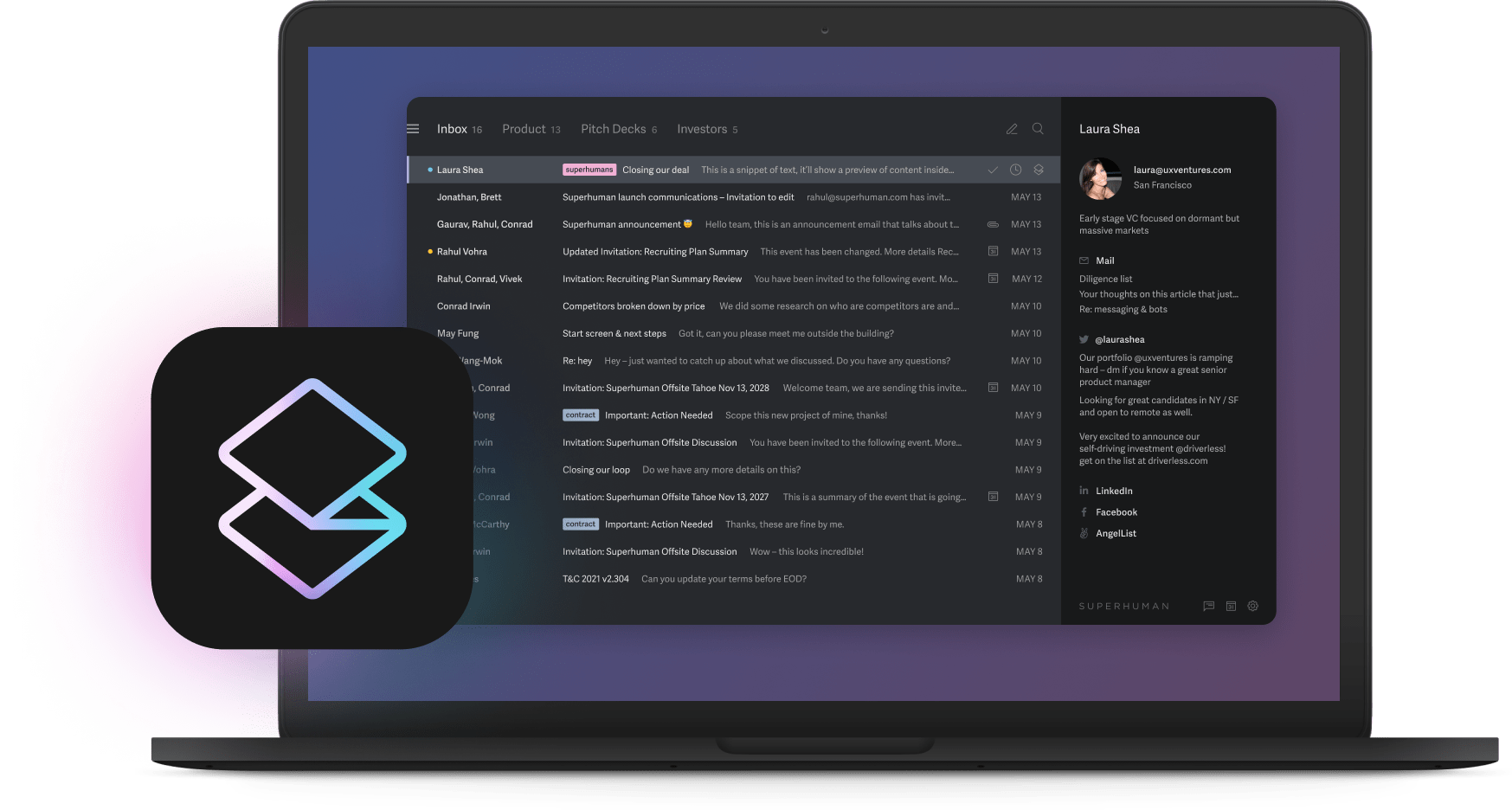