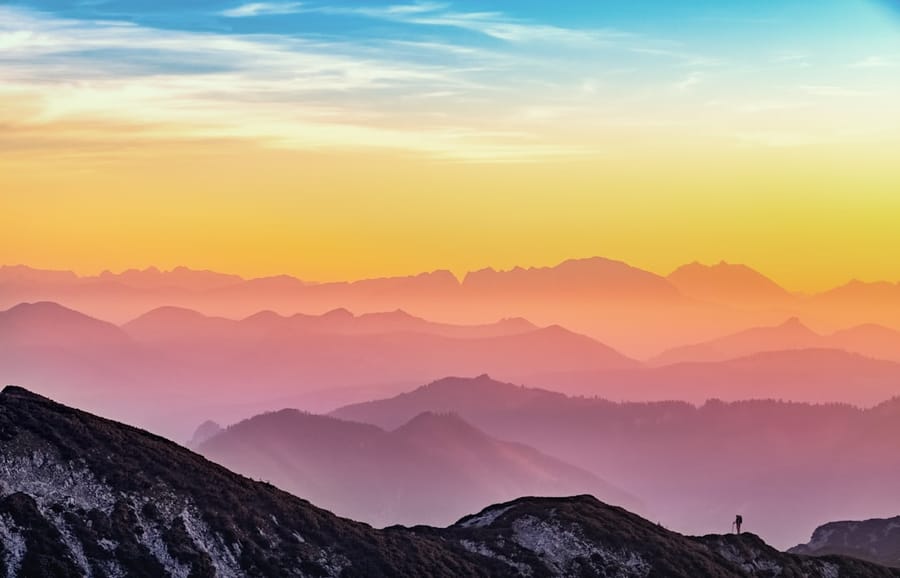
Companies everywhere are finding new ways to work smarter with generative AI. Unlike old-school AI that just analyzes your data, this new tech actually creates stuff on its own — writing emails, designing products, even coming up with business strategies.
The numbers tell the story. Experts predict generative AI will add between $2.6 trillion and $4.4 trillion annually to the global economy. Investors see the gold rush too, pouring over $56 billion into generative AI companies in 2024 alone, almost twice what they invested last year.
For teams running at high speed, this isn't just about small improvements. When you bring generative AI into your workflow, people get more done in less time. The results speak for themselves: worker output jumps by 66% with these tools, and teams save 105 minutes every day.
Let's look at how real companies are making money, saving time, and solving problems with generative AI today.
The 3D approach to generative AI use cases
We've organized these use cases in three dimensions to help you find the right fit for your business:
Dimension 1: Business value drivers
- Cost reduction & efficiency
- Revenue enhancement
- Risk mitigation
- Innovation acceleration
- Customer experience transformation
Dimension 2: Functional applications
- Customer-facing operations
- Internal operations
- Product development
- Strategic decision making
Dimension 3: Implementation complexity
- Quick wins (Low complexity, high impact)
- Strategic investments (Higher complexity, transformative impact)
- Experimental innovations (Higher complexity, uncertain but potentially disruptive impact)
This framework helps you match possible use cases against what matters most to your business. Now let's see exactly how companies are using this technology to get ahead.
Try SuperhumanCustomer-facing operations
1. Quick win: AI-powered customer service
Swedish fintech company Klarna put an AI assistant to work answering customer questions. In just one month, the AI handled two-thirds of all customer chats.
The results? The AI took care of 2.3 million conversations (the work of 700 human agents), customers got answers in under 2 minutes instead of waiting 11 minutes, and repeat questions dropped by 25%.
Implementation approach: Klarna fed the AI thousands of past customer conversations and support documents. They taught it which questions needed a human touch, creating a tag-team system where everyone plays to their strengths.
Business value: Klarna's customer service costs went down, wait times shrank, and human agents now focus on complex issues where empathy matters most.
2. Strategic investment: Personalized marketing at scale
Marketing teams used to spend days creating campaigns for different customer segments. Now they let AI do the heavy lifting. These smart systems now write 30% of marketing materials at many companies, finishing work almost twice as fast while cutting content costs by 30-50%.
When Bayer tried AI for their marketing, clicks jumped 85% year-over-year and they paid 33% less per click. Monks used Google Gemini to help Hatch create ads that got 80% more clicks, 46% more engaged visitors, and cost 31% less per purchase.
Implementation approach: The best companies show the AI their brand voice guide, successful past campaigns, and customer data. The AI learns from these examples and creates personalized content that still sounds like the company.
Business value: More sales through better conversion rates, lower marketing costs, and campaigns that launch in days instead of weeks.
3. Experimental innovation: Digital twin customer experiences
Some pioneering companies now create virtual copies of their customers to test new ideas before rolling them out to real people.
Clothing retailers build models that suggest outfits based on browsing history and social posts. Banks create virtual versions of customer accounts to spot fraud patterns before criminals can strike.
Implementation approach: Companies gather data from every customer touchpoint (website visits, purchase history, service calls) to build detailed profiles that predict what each person will do next.
Business value: You learn what customers want before spending money on new products or experiences, and you catch problems before they affect real customers.
Internal operations
4. Quick win: Automated report generation
Banks and financial companies hate paperwork as much as the rest of us. HSBC now uses AI to write compliance documents that would take humans hours or days to complete.
JP Morgan Chase built an AI system that watches transactions in real time to catch fraud the moment it happens, without accidentally flagging legitimate customer purchases.
Implementation approach: These companies feed the AI thousands of past reports, regulatory requirements, and financial rules. The AI learns the patterns and can produce accurate new documents in minutes.
Business value: Banks save millions in labor costs, make fewer mistakes on critical documents, and free up their experts to solve problems instead of filling out forms.
5. Strategic investment: AI-enhanced knowledge management
Morgan Stanley's wealth managers used to waste hours hunting for information buried in documents. Now they ask an AI assistant instead. 98% of their advisor teams now use this tool, and access to critical information jumped from 20% to 80% of documents.
Implementation approach: They gave the AI access to all their research reports, product details, and internal documentation. Now advisors can ask questions in plain English and get answers in seconds.
Business value: Advisors spend more time with clients and less time searching for information. They make better recommendations because they can actually find and use all the company's knowledge.
6. Experimental innovation: Autonomous process optimization
Forward-thinking companies are teaching AI to find and fix problems in their supply chains without human involvement. These systems constantly watch for bottlenecks, analyze shipping patterns, and predict future problems before they happen.
Implementation approach: The AI studies how work flows through a company by examining every step in processes like order fulfillment or manufacturing. It spots inefficiencies humans might miss and suggests better approaches.
Business value: Problems get fixed before they cost money, processes keep improving without requiring constant human attention, and operations adapt quickly when conditions change.
Product development
7. Quick win: AI-assisted design
Siemens engineers used to spend weeks trying different designs. Now they tell AI what they need, and it creates dozens of options overnight. Their redesigned parts are 75% lighter and they finish projects 30% faster. Companies using their Senseye system have cut maintenance costs by 40%, made maintenance teams 55% more productive, and reduced machine downtime by 50%.
General Electric uses similar technology to predict exactly when equipment will break so they can fix it during planned maintenance instead of after an expensive failure.
Implementation approach: Engineers tell the AI what a product needs to do and what constraints exist (materials, weight, cost). The AI explores thousands of possible designs that humans might never think of.
Business value: Products get to market faster, use less material, perform better, and cost less to build and maintain.
8. Strategic investment: Predictive maintenance systems
Shell got tired of equipment breaking at the worst possible time. Their solution? An AI system that watches 10,000 pieces of equipment around the world, processing data from 3 million sensors. The system makes 15 million predictions daily about what might break next.
Implementation approach: The AI studies years of maintenance records and learns subtle patterns that precede failures. It notices when equipment starts behaving differently, even in tiny ways humans can't detect.
Business value: Shell avoids emergency repair costs, prevents production shutdowns that could cost millions per day, and makes equipment last years longer than expected.
9. Experimental innovation: Generative product customization
Ferrari customers used to wade through complicated option lists. Now Ferrari's AI helps them build their dream car visually. The system has brought in more potential buyers and cut configuration time by 20% by showing people realistic 3D images of their custom designs.
Implementation approach: The AI learns from choices customers have made in the past and current design trends. It suggests combinations that work well together while respecting what's actually possible to build.
Business value: Customers happily pay more for personalized products, feel more satisfied with their choices, and make purchasing decisions faster.
Strategic decision making
10. Quick win: Market intelligence synthesis
Investment firms need to monitor mountains of information about markets, competitors, and economic trends. Now they use AI to read everything and spot important patterns humans might miss. These systems help with risk assessment, trading strategies, and investment decisions by connecting dots across huge datasets.
Implementation approach: The AI reads news articles, earnings reports, economic data, and social media to spot emerging trends or warning signs before they become obvious to everyone else.
Business value: Companies make better decisions faster and spot opportunities or threats before competitors do.
11. Strategic investment: Scenario planning and simulation
Energy companies face complex planning challenges with huge financial stakes. They now use AI to simulate thousands of "what if" scenarios. These systems create realistic simulations of future conditions to help with demand forecasting, infrastructure planning, and emergency response.
Implementation approach: The AI combines historical data with current trends to generate plausible future scenarios. It considers how market conditions, regulations, technology changes, and other factors might interact.
Business value: Companies make better long-term bets on infrastructure and investments by preparing for multiple possible futures instead of just one prediction.
12. Experimental innovation: Autonomous strategy development
The most cutting-edge companies now let AI help shape their business strategy. These systems analyze mountains of data to suggest new market opportunities, competitive responses, and innovative business approaches.
People who incorporate AI into strategy work are 1.8 times more likely to be seen as innovative by their teammates.
Implementation approach: The AI examines company goals, market data, competitive intelligence, and past performance. It suggests new approaches that human teams might miss because of biases or blind spots.
Business value: Companies discover non-obvious ways to grow, avoid competitive threats, and adapt faster to market changes.
Implementation roadmap
Getting generative AI to work in your business doesn't have to be complicated. Here's a straightforward approach that focuses on quick wins and practical results.
Assessment phase: Identify your best opportunities
Start by finding the low-hanging fruit:
- Look at repetitive communication tasks: What emails, customer interactions, or documents does your team write over and over? These patterns are perfect for AI to handle.
- Check your data readiness: Take stock of what you have:
- How many past messages or documents could you use for examples?
- Can you easily access your knowledge base?
- Will your current systems play nice with new AI tools?
- Calculate potential ROI simply:
- Time saved = (Minutes per task × Tasks per week × Number of employees) × Hourly cost
- Response speed = Current response time ÷ Expected AI-assisted time
- Error reduction = (Current error rate − Expected AI error rate) × Cost per error
Pilot phase: Start small, prove value
Pick one focused project that can show clear benefits fast:
Tech stack reality check:
- Look for AI tools that connect to what you already use
- Make sure they work with your:
- CRM (Salesforce, HubSpot)
- Project tools (Asana, Monday)
- Team chat (Slack, Teams)
Skills you'll need:
- Someone who can connect your data sources
- Someone who knows how to write effective AI prompts
- Someone who can make the AI easy for everyone to use
If you don't have these skills in-house, you can:
- Partner with specialists
- Train your current team
- Make targeted hires
Scaling phase: Expand what works
Once your pilot shows results, build on that success:
Simple decision guide:
To pick your next AI project:
- What's your biggest pain point?
- Too many costs → Try automated reporting or AI customer service
- Need more sales → Look at personalized marketing or product customization
- Too slow → Consider knowledge tools or AI design help
- Need fresh ideas → Explore scenario planning or strategy tools
- What tech resources do you have?
- Limited tech team → Stick with packaged solutions
- Strong data team → Build custom implementations
- Advanced AI skills → Try cutting-edge applications
- Which department needs the most help?
- Customer teams → AI customer service or marketing
- Operations → Knowledge systems or report automation
- Product teams → AI design tools or predictive maintenance
- Strategy → Market intelligence or scenario planning
Economic research suggests these tools could increase worker productivity by 0.1-0.6% annually through 2040. For companies that move quickly, this means a real advantage over slower competitors. for critical capabilities
Scaling phase: Expand and integrate
As pilots show value, expand to more strategic investments:
Implementation decision tree:
To figure out your next generative AI implementation:
- What's your primary business goal?
- Reduce costs → Start with automated reporting or customer service AI
- Increase revenue → Implement personalized marketing or product customization
- Improve speed → Deploy knowledge management or AI-assisted design
- Enhance innovation → Explore scenario planning or strategy development
- What's your current AI readiness?
- Limited data or technical resources → Focus on quick wins with packaged solutions
- Strong data infrastructure → Pursue strategic investments with custom implementations
- Advanced AI capabilities → Experiment with cutting-edge applications
- Which functional area needs most improvement?
- Customer-facing operations → AI-powered customer service or marketing
- Internal operations → Knowledge management or report automation
- Product development → AI-assisted design or predictive maintenance
- Strategic decision-making → Market intelligence or scenario planning
Economic research suggests generative AI could boost labor productivity by 0.1-0.6% annually through 2040. For fast-moving companies that implement early and effectively, the competitive advantages will be substantial and lasting. of employees) × Hourly cost
- Response improvement = Current average response time ÷ Expected AI-assisted response time
- Error reduction = (Current error rate − Expected AI error rate) × Cost per error
Pilot phase: Start small, prove value
Choose a focused implementation that can demonstrate clear benefits:
Tech stack integration considerations:
- Prioritize solutions with APIs that connect to your existing tools
- Evaluate generative AI platforms that integrate with your:
- CRM systems (Salesforce, HubSpot)
- Project management tools (Asana, Monday)
- Communication platforms (Slack, Teams)
- Email systems
Skills assessment checklist:
- Technical capabilities needed:
- Data engineering (connecting data sources)
- Prompt engineering (optimizing AI instructions)
- User experience design (creating intuitive interfaces)
- If gaps exist, consider:
- Partnerships with specialized AI implementation firms
- Targeted technical training for existing team members
- Strategic hiring for critical capabilities
Scaling phase: Expand and integrate
As pilots demonstrate value, expand to more strategic investments:
Implementation decision tree:
To determine your next generative AI implementation:
- What's your primary business goal?
- Reduce costs → Start with automated reporting or customer service AI
- Increase revenue → Implement personalized marketing or product customization
- Improve speed → Deploy knowledge management or AI-assisted design
- Enhance innovation → Explore scenario planning or strategy development
- What's your current AI readiness?
- Limited data or technical resources → Focus on quick wins with packaged solutions
- Strong data infrastructure → Pursue strategic investments with custom implementations
- Advanced AI capabilities → Experiment with cutting-edge applications
- Which functional area needs most improvement?
- Customer-facing operations → AI-powered customer service or marketing
- Internal operations → Knowledge management or report automation
- Product development → AI-assisted design or predictive maintenance
- Strategic decision-making → Market intelligence or scenario planning
Economic research suggests generative AI could enable labor productivity growth of 0.1-0.6% annually through 2040. For fast-moving companies that implement early and effectively, the competitive advantages will be substantial and lasting.
The bottom line
Companies that get the most from generative AI focus on practical problems first, not flashy technology. They make sure they have clean, usable data and recognize that success requires both technical know-how and thoughtful people management.
The question isn't whether to use these tools, but how quickly you can get them working on your biggest challenges to gain real advantages in efficiency, revenue, and customer experience.
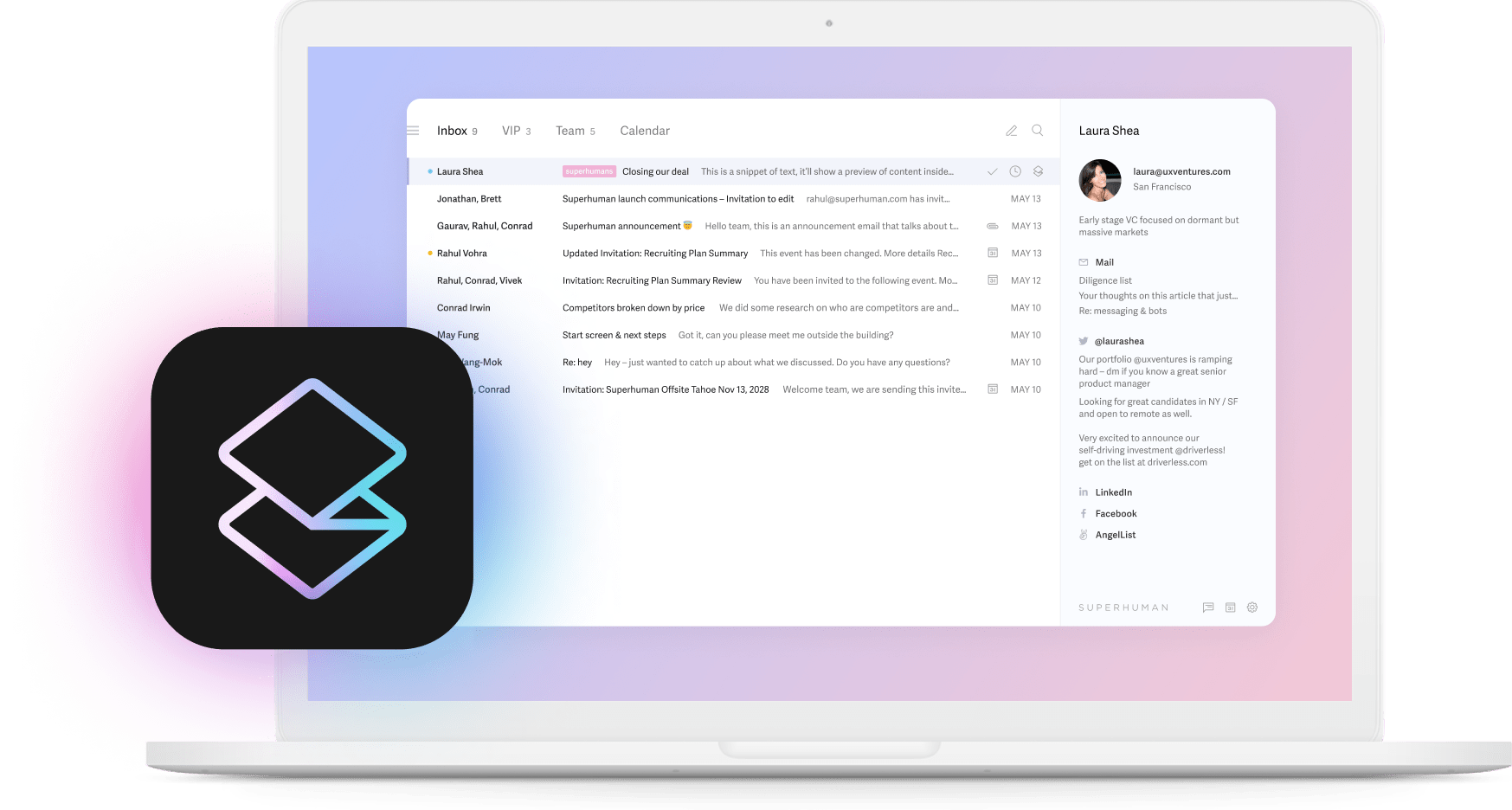
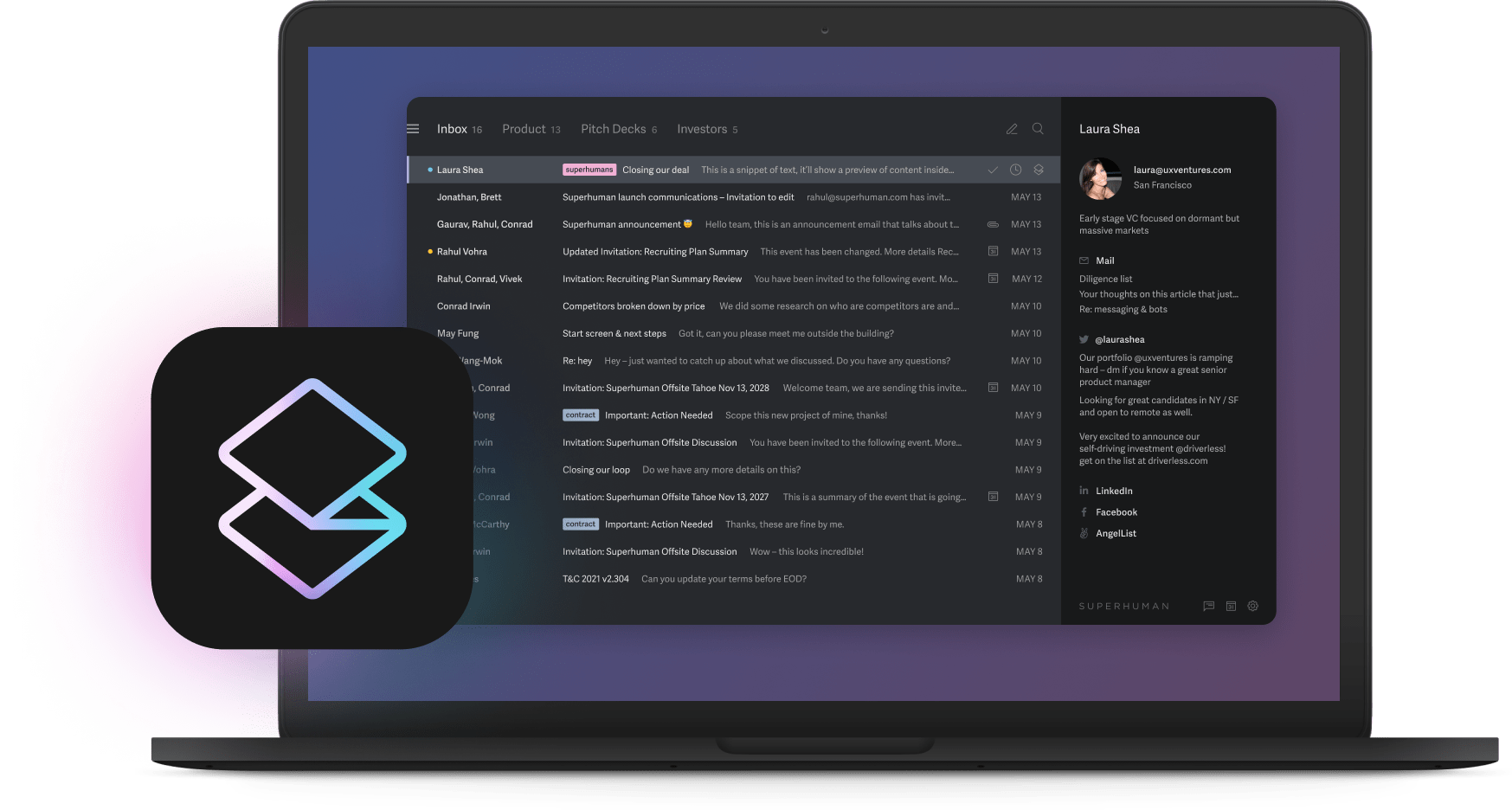